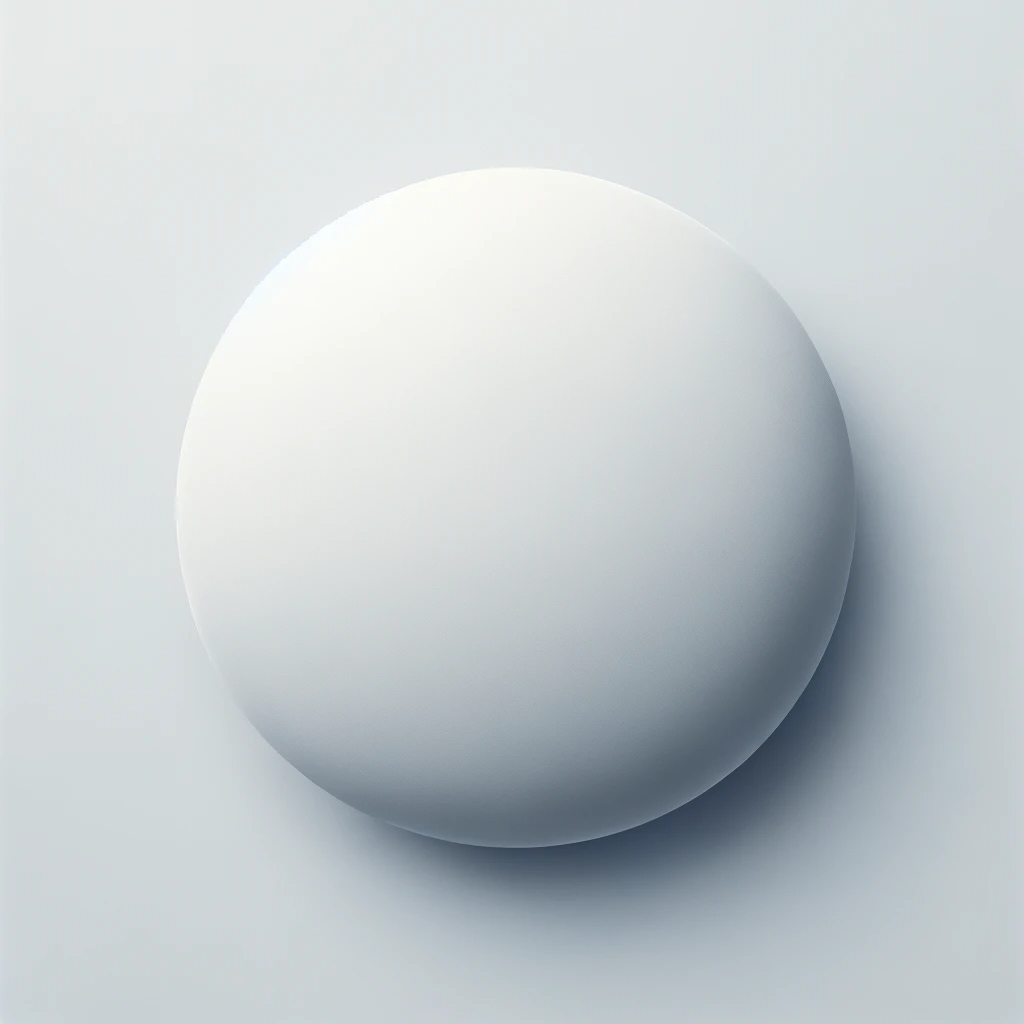
In this post, we introduce GANSynth, a method for generating high-fidelity audio with Generative Adversarial Networks (GANs). ... @inproceedings{gansynth, title = {GANSynth: Adversarial Neural Audio Synthesis}, author = {Jesse Engel and Kumar Krishna Agrawal and Shuo Chen and Ishaan …Jul 18, 2022 · Introduction. Generative adversarial networks (GANs) are an exciting recent innovation in machine learning. GANs are generative models: they create new data instances that resemble your training data. For example, GANs can create images that look like photographs of human faces, even though the faces don't belong to any real person. Verizon has been at the forefront of the 5G revolution, offering its customers lightning-fast internet speeds and low latency. Verizon’s 5G network is the fifth generation of wirel...erate adversarial examples for the testing of these new semantics based approaches. The model defined in this paper is a step towards such a generator, building on the work of …Generative adversarial networks (GANs) have seen remarkable progress in recent years. They are used as generative models for all kinds of data such as text, images, audio, music, videos, and animations. This paper presents a comprehensive review of the novel and emerging GAN-based speech frameworks …Jan 7, 2018 · Generative Adversarial Network framework. GANs are generative models devised by Goodfellow et al. in 2014. In a GAN setup, two differentiable functions, represented by neural networks, are locked in a game. The two players (the generator and the discriminator) have different roles in this framework. Oct 3, 2022 · A generative adversarial network, constrained by the sum of global precipitation, is developed that substantially improves ESM predictions of spatial patterns and intermittency of daily precipitation. Jul 6, 2019 ... The generative model tries to predict features given classes. This involves determining the probability of a feature given a class. It acts as a ...We address this by employing a cycle generative adversarial network (CycleGAN) with a reciprocal space discriminator, which augments simulated data with realistic spatial frequency information.See full list on machinelearningmastery.com OpenAI. You have now coded the majority of your network. All that remains is to train this network and take a look at the images that you created. def train( epochs =1, batch_size =128): # Get the training and testing data. x_train, y_train, x_test, y_test = load_minst_data () # Split the training data into batches of size 128. In this study, we introduce omicsGAN, a generative adversarial network model to integrate two omics data and their interaction network. The model captures information from the interaction network as well as the two omics datasets and fuse them to generate synthetic data with better predictive signals. Results: Large-scale experiments …Generative adversarial networks (GANs) present a way to learn deep representations without extensively annotated training data. These networks achieve learning through deriving back propagation signals through a competitive process involving a pair of networks. The representations that can be learned by GANs may be used in …Generative adversarial network (GAN) is a famous deep generative prototypical that effectively makes adversarial alterations among pairs of neural networks. GAN generally attempts to plot a sample z from a previous distribution p(z) to the data-space. However, the discriminatory net attempts to calculate the likelihood where input is an actual ...A generative adversarial network, constrained by the sum of global precipitation, is developed that substantially improves ESM predictions of spatial patterns and intermittency of daily precipitation.Two popular approaches are GANs, which are used to generate multimedia, and VAEs, used more for signal analysis. Generative adversarial networks and variational autoencoders are two of the most popular approaches used for producing AI-generated content. In general, GANs tend to be more widely …Social GAN: Socially Acceptable Trajectories with Generative Adversarial Networks. Understanding human motion behavior is critical for autonomous moving platforms (like self-driving cars and social robots) if they are to navigate human-centric environments. This is challenging because human motion …Adventure sports clubs and networks let you share your passion for adventure. Visit HowStuffWorks to learn about adventure sports clubs and networks. Advertisement Adventure sports...Compared to these methods, our proposed method first exploits the generator network to generate clear and fine faces with high resolution (\(4\times \) up-scaling), and then the discriminator network is trained to distinguish the target faces from the input images.2.3 Generative Adversarial Networks. In the seminal work …Generative adversarial networks (GANs) are among the most versatile kinds of AI model architectures, and they're constantly improving.In recent years, the rollout of 5G technology has been making waves across the globe. With its promise of faster speeds, lower latency, and a more connected world, it’s no wonder t...Recently, there have been claims that generative models such as generative adversarial networks (GANs) can synthesize MR contrasts that are not acquired. However, the poor scalability of existing ...Recent studies have shown remarkable success in image-to-image translation for two domains. However, existing approaches have limited scalability and robustness in handling more than two domains, since different models should be built independently for every pair of image domains. To address this limitation, we …Generative adversarial networks (GANs) 8,9,10,11,12,13 are a new type of generative model and aim to generate high-quality synthetic samples by accurately learning the underlying distributions of ...In this paper, we propose AdvGAN to generate adversarial examples with generative adversarial networks (GANs), which can learn and approximate the distribution of original instances. For AdvGAN, once the generator is trained, it can generate adversarial perturbations efficiently for any instance, so as to potentially …Generative adversarial network (GAN) studies have grown exponentially in the past few years. Their impact has been seen mainly in the computer vision field with realistic image and video manipulation, especially generation, making significant advancements. Although these computer vision advances have garnered much …With the advent of 5G technology, people around the world are eagerly anticipating the lightning-fast speeds and low latency that this next-generation network promises to deliver. ...Followed by the early attempts using deep convolutional neural networks (CNNs) [8,9], generative adversarial networks (GANs) that consist of two CNN networks—one generator and one discriminator—have been demonstrated to exhibit better performance on nonlinear intensity transformation between source and target images …The ideal loss curves for a generative adversarial network (GAN) is shown in Fig. 1D, in which the network reaches the Nash equilibrium. For training, we use the fundus and angiography data-set ...Learn what a GAN is, how it works, and what are its use cases. A GAN is a deep learning architecture that trains two neural networks to compete and generate new data from a …Generative Adversarial Networks (GANs) can generate synthetic animal data by learning from the legacy animal study results, thus may serve as an alternative approach to assess untested chemicals ...Jun 23, 2020 · A generative adversarial network (GAN) is a powerful approach to machine learning (ML). At a high level, a GAN is simply two neural networks that feed into each other. One produces increasingly accurate data while the other gradually improves its ability to classify such data. In this blog we’ll dive a bit deeper into how this mechanism works ... Generative adversarial networks has been sometimes confused with the related concept of “adversar-ial examples” [28]. Adversarial examples are examples found by using gradient-based optimization directly on the input to a classification network, in order to find examples that are similar to the data yet misclassified. The Super-Resolution Generative Adversarial Network (SRGAN) is a seminal work that is capable of generating realistic textures during single image super-resolution. However, the hallucinated details are often accompanied with unpleasant artifacts. To further enhance the visual quality, we thoroughly study …Attentive Generative Adversarial Network for Raindrop Removal from a Single Image. Raindrops adhered to a glass window or camera lens can severely hamper the visibility of a background scene and degrade an image considerably. In this paper, we address the problem by visually removing raindrops, and thus transforming a raindrop …Learn what a GAN is, how it works, and what are its use cases. A GAN is a deep learning architecture that trains two neural networks to compete and generate new data from a … GANs are a class of density-free generative models with (mostly) unrestricted generator functions. Introducing adversial discriminator networks allows GANs to learn by minimizing the Jensen-Shannon divergence. Concurrently learning the generator and discriminator is challenging due to. Intro to Generative Adversarial Networks (GANs) by Margaret Maynard-Reid on September 13, 2021. This post covers the intuition of Generative Adversarial Networks (GANs) at a high level, the various GAN variants, and applications for solving real-world problems. This is the first post of a GAN tutorial …The Super-Resolution Generative Adversarial Network (SRGAN) is a seminal work that is capable of generating realistic textures during single image super-resolution. However, the hallucinated details are often accompanied with unpleasant artifacts. To further enhance the visual quality, we thoroughly study …Jun 10, 2014 · Feb 2024. Generative models are built using an adversarial process that combines two models. A generator that predicts the data distribution and a Discriminator that analyzes the likelihood that a ... Generative Adversarial Networks (GANs) can generate synthetic animal data by learning from the legacy animal study results, thus may serve as an alternative approach to assess untested chemicals ...Two popular approaches are GANs, which are used to generate multimedia, and VAEs, used more for signal analysis. Generative adversarial networks and variational autoencoders are two of the most popular approaches used for producing AI-generated content. In general, GANs tend to be more widely …Network security is the combination of policies and procedures implemented by a network administrator to avoid and keep track of unauthorized access, exploitation, modification or ...The ideal loss curves for a generative adversarial network (GAN) is shown in Fig. 1D, in which the network reaches the Nash equilibrium. For training, we use the fundus and angiography data-set ...With the rapid advancements in technology, the rollout of 5G networks has become a hot topic of discussion. As more and more devices become compatible with this new generation of w...Security and privacy are big concerns these days, particularly when it comes to dealing with sensitive information on the internet. Interested in maintaining your anonymity online?...Jul 18, 2022 · Introduction. Generative adversarial networks (GANs) are an exciting recent innovation in machine learning. GANs are generative models: they create new data instances that resemble your training data. For example, GANs can create images that look like photographs of human faces, even though the faces don't belong to any real person. Aug 3, 2022 · A generative adversarial network, or GAN, is a deep neural network framework that can learn from training data and generate new data with the same characteristics as the training data. For example, generative networks trained on photographs of human faces can generate realistic-looking faces which are entirely fictitious. Jan 10, 2018 · Generative adversarial networks (GANs) provide a way to learn deep representations without extensively annotated training data. They achieve this by deriving backpropagation signals through a competitive process involving a pair of networks. The representations that can be learned by GANs may be used in a variety of applications, including image synthesis, semantic image editing, style ... Learn about the basics, components, and optimization of GANs, a type of neural network that can generate realistic images. See examples of GAN models and …Generative adversarial networks (GANs) have been advancing and gaining tremendous interests from both academia and industry. With the development of wireless technologies, a huge amount of data generated at the network edge provides an unprecedented opportunity to develop GANs …Generative Adversarial Networks (GANs) were developed in 2014 by Ian Goodfellow and his teammates. GAN is basically an approach to generative modeling that generates a new set of data based on training data that look like training data. GANs have two main blocks (two neural networks) which compete …Jan 20, 2020 · Generative adversarial networks (GANs) are a hot research topic recently. GANs have been widely studied since 2014, and a large number of algorithms have been proposed. However, there is few comprehensive study explaining the connections among different GANs variants, and how they have evolved. In this paper, we attempt to provide a review on various GANs methods from the perspectives of ... In today’s digital age, where online security threats are prevalent, creating strong and secure passwords is of utmost importance. One effective way to ensure the strength of your ...Generative adversarial networks (GANs) are a hot research topic recently. GANs have been widely studied since 2014, and a large number of algorithms have been proposed. However, there is few comprehensive study explaining the connections among different GANs variants, and how they have …Recently, 5G has started taking the world by storm. But just how does it differ from 4G? The superfast fifth-generation mobile network, most commonly referred to as 5G, is a mobile...In this work a Generative Adversarial approach has been taken to do speech enhancement (i.e. removing noise from corrupted speech signals) with a fully convolutional architecture schematized as follows: This model deals with raw speech waveforms on many noise conditions at different SNRs (40 at training time and 20 during test).Nov 12, 2017 · Data Augmentation Generative Adversarial Networks. Effective training of neural networks requires much data. In the low-data regime, parameters are underdetermined, and learnt networks generalise poorly. Data Augmentation alleviates this by using existing data more effectively. However standard data augmentation produces only limited plausible ... Nov 12, 2017 · Data Augmentation Generative Adversarial Networks. Effective training of neural networks requires much data. In the low-data regime, parameters are underdetermined, and learnt networks generalise poorly. Data Augmentation alleviates this by using existing data more effectively. However standard data augmentation produces only limited plausible ... Generative adversarial networks (GANs) are among the most versatile kinds of AI model architectures, and they're constantly improving.Jul 18, 2022 · Introduction. Generative adversarial networks (GANs) are an exciting recent innovation in machine learning. GANs are generative models: they create new data instances that resemble your training data. For example, GANs can create images that look like photographs of human faces, even though the faces don't belong to any real person. GANs are a class of density-free generative models with (mostly) unrestricted generator functions. Introducing adversial discriminator networks allows GANs to learn by minimizing the Jensen-Shannon divergence. Concurrently learning the generator and discriminator is challenging due to.A generator has lots of uses around the home so working out exactly what you need one for will help you pick the right one. Portable generators do a great job particularly if you o...Learn how GANs work by building the reasoning step by step from the basics of random variable generation. Discover the architecture, the loss function and the …Download a PDF of the paper titled Efficient Geometry-aware 3D Generative Adversarial Networks, by Eric R. Chan and 10 other authors. Unsupervised generation of high-quality multi-view-consistent images and 3D shapes using only collections of single-view 2D photographs has been a long-standing …When it comes to purchasing a generator, one of the first decisions you’ll need to make is whether to buy a new one or opt for a used generator. Both options have their own advanta...Oct 25, 2020 ... GANs [1] introduce the concept of adversarial learning, as they lie in the rivalry between two neural networks. These techniques have enabled ...Abstract—Generative adversarial networks (GANs) are a hot research topic recently. GANs have been widely studied since 2014, and a large number of algorithms have been proposed. However, there is few comprehensive study explaining the connections among different GANs variants, and how they have evolved.With the advent of 5G technology, people around the world are eagerly anticipating the lightning-fast speeds and low latency that this next-generation network promises to deliver. ...Generative adversarial networks have been widely applied in image-to-image translation, since the generator in GAN can generate images with excellent visual properties. The conditional generative adversarial network (cGAN) is a widely used framework for image-to-image translation tasks due to its ability to …We introduce a new algorithm named WGAN, an alternative to traditional GAN training. In this new model, we show that we can improve the stability of learning, get rid of problems like mode collapse, and provide meaningful learning curves useful for debugging and hyperparameter searches. Furthermore, we …Advertisement The smart grid uses computer technology to improve the communication, automation, and connectivity of the various components of the power network. This allows -- as a...Generative Adversarial Nets Ian J. Goodfellow, Jean Pouget-Abadie, Mehdi Mirza, Bing Xu, David Warde-Farley, Sherjil Ozairy, Aaron Courville, Yoshua Bengio z ... Deep belief networks (DBNs) [16] are hybrid models containing a single undirected layer and sev-eral directed layers. While a fast approximate layer-wise training criterion exists ...The Super-Resolution Generative Adversarial Network (SR- GAN) is a seminal work that is capable of generating realistic textures during single image super-resolution. However, the hallucinated details are often accompanied with unpleasant artifacts.Generative Adversarial Networks (GANs) can generate synthetic animal data by learning from the legacy animal study results, thus may serve as an alternative approach to assess untested chemicals ...Generative Adversarial Networks for Markovian Temporal Dynamics: Stochastic Continuous Data GenerationSung Woo Park, Dong Wook Shu, Junseok KwonIn ...Jan 10, 2018 · Generative adversarial networks (GANs) provide a way to learn deep representations without extensively annotated training data. They achieve this by deriving backpropagation signals through a competitive process involving a pair of networks. The representations that can be learned by GANs may be used in a variety of applications, including image synthesis, semantic image editing, style ... The numerical results demonstrated that the proposed ST-EGAN can reduce the mean rmse by 4.78% compared to interpolation algorithms, and reduce the rmse by 0.14% and 0.21% compared with deep convolutional generative adversarial networks and super-resolution convolutional networks, respectively, in the presence of noises with …Nov 11, 2021 · Learn more about watsonx: https://ibm.biz/BdvxDJGenerative Adversarial Networks (GANs) pit two different deep learning models against each other in a game. I... Recent studies have shown remarkable success in image-to-image translation for two domains. However, existing approaches have limited scalability and robustness in handling more than two domains, since different models should be built independently for every pair of image domains. To address this limitation, we …We tackle this problem by combining tools from sequence prediction and generative adversarial networks: a recurrent sequence-to-sequence model observes motion histories and predicts future behavior, using a novel pooling mechanism to aggregate information across people. We predict socially plausible futures by training …Recently, 5G has started taking the world by storm. But just how does it differ from 4G? The superfast fifth-generation mobile network, most commonly referred to as 5G, is a mobile...A generative adversarial network (GAN)-based vocoder trained with an adversarial discriminator is commonly used for speech synthesis because of its fast, …A Generative Adversarial Network (GAN) emanates in the category of Machine Learning (ML) frameworks. These networks have acquired their inspiration …We introduce a new algorithm named WGAN, an alternative to traditional GAN training. In this new model, we show that we can improve the stability of learning, get rid of problems like mode collapse, and provide meaningful learning curves useful for debugging and hyperparameter searches. Furthermore, we …Generative Adversarial Networks (GAN) have received wide attention in the machine learning field for their potential to learn high-dimensional, complex real data distribution. Specifically, they do not rely on any assumptions about the distribution and can generate real-like samples from latent space in a …. The main contributions of this work are: We prTwo popular approaches are GANs, which are In today’s digital age, where online security threats are prevalent, creating strong and secure passwords is of utmost importance. One effective way to ensure the strength of your ...Jan 7, 2018 ... Generative Adversarial Networks · The generator trying to maximize the probability of making the discriminator mistakes its inputs as real. Learn about generative models, a branch of unsuperv Generative Adversarial Networks. Modeling artificial samples after a given dataset can be done directly by comparing the true data with the generated data, or indirectly by utilizing a downstream task that in turn enables the network to generate real samples. For direct approaches, one can use the Maximum Mean … The ideal loss curves for a generative adversarial network (GAN) ...
Continue Reading